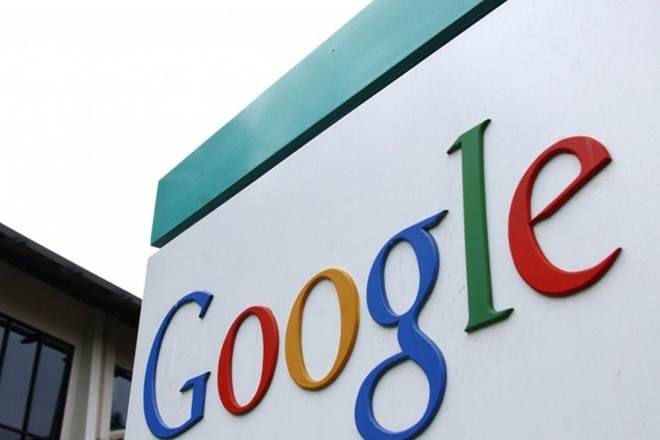
In a landmark breakthrough, Google has announced the development of artificial intelligence system that can be used to detect breast cancer more effectively than doctors would.
The system was developed through collaboration between Google and researchers, and has been proved successful according to Scientific Journal Nature where it was published on Wednesday.
Using the AI technology resulted in more accurate detection of cancer cells in the body, reducing cases of false results significantly. Health workers have had to deal with cases where test results say positive when it is negative or negative when it is positive. The program was trained to detect tens of thousands of mammograms from 25,856 women in the UK and 3,097 women in the US. The system was then used to identify the presence of breast cancer in mammograms of women who were known to have either biopsy-proven breast cancer or normal follow up imaging results at least 365 days later.
Tekedia Mini-MBA edition 14 (June 3 – Sept 2, 2024) begins registrations; get massive discounts with early registration here.
Tekedia AI in Business Masterclass opens registrations here.
Join Tekedia Capital Syndicate and invest in Africa’s finest startups here.
Early results show improvement in cancer detection compared to human experts. McKinney and colleagues, the authors of the programme report that the AI system outperformed both the historical decisions made by the radiologists who initially assessed mammograms, and the decisions of 6 expert radiologists who interpreted 500 randomly selected cases in a controlled study.
The program is part of series of studies investigating the use of artificial intelligence in a range of medical-imaging contexts.
The system was more accurate even though it has no patient’s previous health information to work with it. Compared to human experts, the program reduced false positives by 5.7% for US women and 12% for UK women. It reduced false negatives by 9.4% for US women and 2.7% for UK women.
Breast cancer screening programmes routinely measure their diagnostic performance – whether cancer is correctly detected (true positive) or missed (false positive). Some areas marked on mammograms might be identified as abnormal but turn out not to be cancerous (false Positive) upon further testing.
While the development beckons hope of future where AI would be relied on for early detection of cancer, there is a need to develop the system to accommodate medical diversity among other issues. The authors note that clinical trials will be needed to further assess the utility of this tool in medical practice. The research has been centered on a controlled environment which covers a little percent of varying world cancer cases.
For instance, the study did not include all the different mammography technologies currently in use, and most images were obtained using a mammography system from a single manufacturer. The study included examples of two types of mammogram: Tomosynthesis (3D mammography) and conventional digital (2D) mammography. There is a need to know how each of the systems performed individually for each technology.
Another reason not to celebrate yet is demographics. The demographics studied is only defined by age, and the performance of AI algorithms can be highly dependent on the population used in the training sets. So a representative sample of the general population is needed to be used in the development of the system to ensure that whatever results it yields will be widely applicable.
Another area of challenge comes from lessons learnt from Computer Aided Detection (CAD) of breast cancer. CAD is a computer system developed to interpret mammography in clinics. In the beginning of its experimental testing, it was promising but fell short in real-world settings. According to Nature, CAD marks mammograms to draw the interpreter’s attention to areas that might be abnormal. But analysis of a large sample of clinical mammography interpretations from the US Breast Cancer Surveillance Consortium registry showed that CAD lacks diagnostic accuracy. The study went further to reveal that the programme worsened (sensitivity), the ability of radiologists to determine if cancer is present. So CAD instead of improving cancer detection improved likelihood of false positive result.
So in large scale, CAD failed to deliver the same result it offered during the experimental tests.
But unlike CAD, AI has the ability to learn from the data it acquired in the course of testing, which heightens the hope that science is getting close to beating the hurdles in early cancer detection.
Professor Ara Darzi, one of the authors of the paper and the director of the Cancer Research UK Imperial Center, told CNN that the AI system has been unexpectedly impressive.
“This is one of those transformational discoveries you have in your hand, which could disrupt the way we deliver screening in terms of improving accuracy and productivity,” he said.
While the new AI study beams with hope, harmonized and anonymized data stand in the way of its success if it’s not incorporated into issues needed to be addressed. The Nature concluded the report this way:
“To achieve the promise of AI in health care that is implied by McKinney and colleagues’ study, anonymized data in health records might thus have to be treated as precious resources of potential benefit to human health, in much time the same way as public utilities such as drinking water are currently treated. Clearly, however, if such AI systems are to be developed and used widely, attention must be paid to patient privacy, and to how data are stored and used, by whom, and with what type of oversight.”