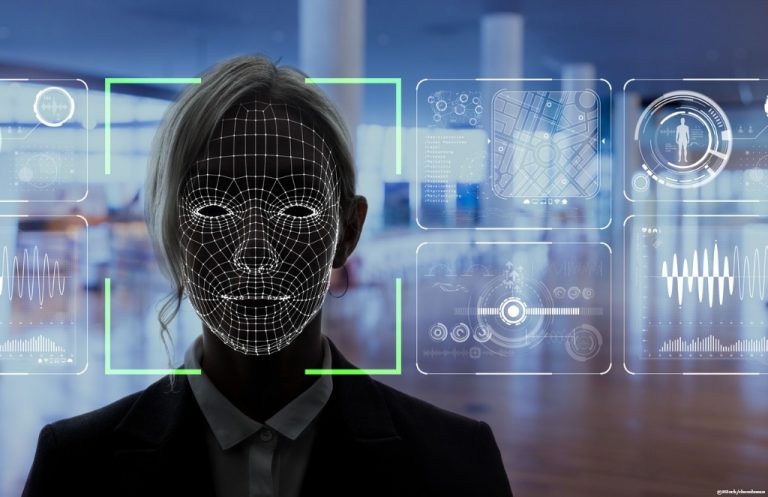
In the past few years, we have witnessed a growing awareness and demand for privacy among consumers, businesses and regulators. Privacy is no longer a niche topic for tech enthusiasts or activists, but a mainstream concern that affects everyone who uses digital products and services.
Privacy is going mainstream for several reasons. First, the increasing frequency and severity of data breaches, hacks and leaks have exposed the risks and harms of sharing personal information online. Second, the proliferation of data-driven technologies such as artificial intelligence, facial recognition and biometrics have raised ethical and legal questions about how our data is collected, used and shared.
Third, the emergence of new privacy laws and regulations such as the General Data Protection Regulation (GDPR) in Europe and the California Consumer Privacy Act (CCPA) in the US have created new rights and obligations for both data subjects and data controllers.
Register for Tekedia Mini-MBA edition 18 (Sep 15 – Dec 6, 2025) today for early bird discounts. Do annual for access to Blucera.com.
Tekedia AI in Business Masterclass opens registrations.
Join Tekedia Capital Syndicate and co-invest in great global startups.
Register for Tekedia AI Lab: From Technical Design to Deployment.
These developments have created new challenges and opportunities for businesses that rely on data as a key asset. On one hand, businesses need to comply with the evolving privacy rules and expectations of their customers, partners and regulators.
On the other hand, businesses can leverage privacy as a competitive advantage and a source of innovation. By adopting privacy-by-design principles, implementing privacy-enhancing technologies and offering privacy-friendly products and services, businesses can build trust, loyalty and differentiation in the market.
Privacy Enhancing Technologies (PETs) are tools and methods that aim to protect the personal data of individuals and organizations from unauthorized access, use, or disclosure. PETs can help users to exercise control over their own data, enhance their trust in online services, and comply with data protection regulations.
There are different types of PETs, such as encryption, anonymization, pseudonymization, data minimization, differential privacy, and zero-knowledge proofs. Each of these techniques has its own advantages and limitations, depending on the context and the goals of the data processing.
Encryption is the process of transforming data into an unreadable form, using a secret key. Only those who have the key can decrypt the data and access its original content. Encryption can protect data in transit (such as when sending an email or browsing a website) or at rest (such as when storing data on a device or a cloud service).
Anonymization is the process of removing or modifying any information that can identify a person or a group of persons from a dataset. Anonymized data cannot be linked back to the original individuals, even with additional information or sophisticated techniques. Anonymization can enable data sharing and analysis for research or statistical purposes, without compromising the privacy of the data subjects.
Pseudonymization is the process of replacing identifying information with artificial identifiers, such as random numbers or codes. Pseudonymized data can still be linked back to the original individuals, but only with access to a separate database that contains the mapping between the identifiers and the real identities. Pseudonymization can reduce the risks of data breaches and unauthorized access, while preserving some functionality and utility of the data.
Data minimization is the principle of collecting and processing only the minimum amount of data that is necessary for a specific purpose. Data minimization can reduce the exposure and impact of potential privacy violations, as well as the costs and complexity of data management. Data minimization can be achieved by applying techniques such as data aggregation, filtering, sampling, or deletion.
Differential privacy is a mathematical framework that quantifies the privacy loss of a data analysis algorithm. Differential privacy guarantees that the output of an algorithm does not reveal much information about any individual in the dataset, regardless of what other information is available. Differential privacy can enable privacy-preserving data analysis and machine learning, while providing rigorous and provable guarantees.
Zero-knowledge proofs are cryptographic protocols that allow one party to prove to another party that a certain statement is true, without revealing any other information. Zero-knowledge proofs can enable secure authentication, verification, and computation on sensitive data, without disclosing the data itself.
PETs are not only technical solutions, but also social and legal ones. PETs require the collaboration and coordination of various stakeholders, such as users, developers, providers, regulators, and researchers. PETs also need to comply with ethical principles and legal frameworks, such as the General Data Protection Regulation (GDPR) in the European Union.
PETs are not a panacea for privacy challenges, but rather a part of a holistic approach that involves awareness, education, empowerment, and accountability. PETs can help users to protect their privacy rights and interests, but they also come with responsibilities and trade-offs. Users need to understand how PETs work, what benefits and risks they entail, and how to use them effectively and appropriately.