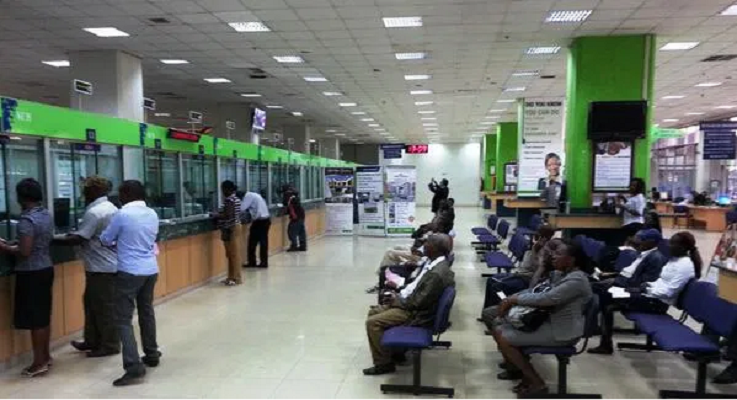
I had an idea in 2013 to help improve, optimize or even eliminate queues in banking halls. It was about two years into my career as a HR professional. It is now about 10 years into my career and I still believe in the idea.
I first came across the concept of queuing theory in 2013 and its applications to banking were self-evident. I tried to conceptualize solving virtual queues with the theory_ imaginary queues like number of persons on a “promotion queue” as well as number of persons waiting to go on leave to mention a few. I shared my cogitations with my then team members. It was a wonderful presentation. But then it ended at that – a wonderful presentation, nothing more.
I was young in my career, so obviously, I had little clout to pursue my theories to a logical conclusion, albeit if implemented would have been one of those innovations that could have caused an inflection point in Banking
Register for Tekedia Mini-MBA edition 18 (Sep 15 – Dec 6, 2025) today for early bird discounts. Do annual for access to Blucera.com.
Tekedia AI in Business Masterclass opens registrations.
Join Tekedia Capital Syndicate and co-invest in great global startups.
Register to become a better CEO or Director with Tekedia CEO & Director Program.
I would walk you through the conceptual solution to the queuing challenge I formulated in 2013. But before then let me lay a background to queuing theory.
Queuing Theory
Queuing theory is “a mathematical study of congestion and delays of waiting in line. It is a discipline in Operations Research that examines every component of waiting in line to be served, including the arrival process, service process, number of servers, number of system places, and the number of customers”
Two important metrics in studying any kind of queue (or waiting) is the mean arrival rate (mAR) and mean service rate(mSR). A queue will always form when the former exceeds the later, i.e mAR > mSR. And there also lies the solution to a queuing challenge: tinkering with mSR.
Now for a Bank with a distributary of branches, (sometimes two less than a km away) while it can not control the arrival rate of customers, it can calculate mAR for every branch. Armed with this metric, it is theoretically possible to reassign tellers and customer service personnel such that mSR will be equal to mAR at the minimum. We all have had experiences where we went into a bank at supposedly peak hour to find the banking hall empty and under-utilized personnel. The answer for Banks is not hiring new staff, it is redistribution of personnel. An action which will be armed with proper analytics of the mAR per branch.
Calculating mAR and mSR for a Bank
With the advances in Machine Learning (ML) and Artificial Intelligence (AI), it is not difficult to conceive how mAR can be calculated for every customer who comes to the branch over a time period. mAR calculations deal with customer arrival patterns and the time difference between the arrival of one customer and the next. mSR calculations deals with the average time it took to service the customer. Most banks already have data on mSR (or at least an approximation of it) without knowing. Most in branch banking transactions are electronically logged e.g. like the time stamp when a teller calls up an account to post on it and when the transaction was successfully posted. The time difference per customer divided by the total number of customers is an approximation of mSR for some class of customers.
For mAR, one can easily train a facial recognition software to recognize a human being at the entrance and log the time of entry between each successive customer. With mAR and mSR, queuing challenges can be optimized in a bank.
Summarizing
While this is a simplistic and high-level solution to the problem, it does reveal that at least there is a conceptual solution to the problem of waiting; not just for banks but for any service industry. And about the same conceptual thinking can be adopted to solve the problem.