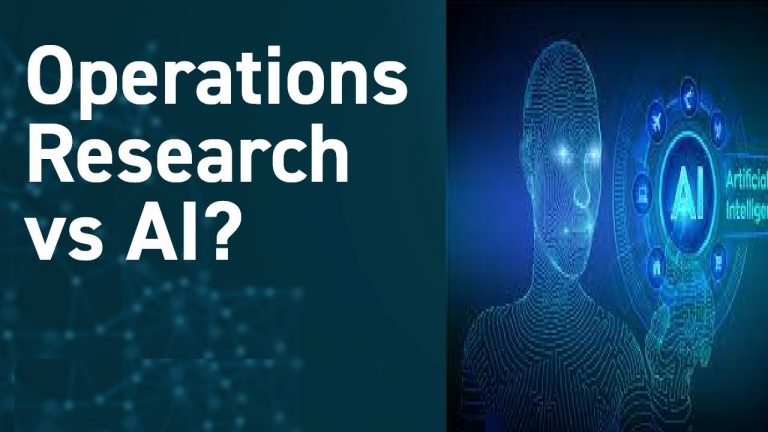
Modern problems need modern solutions. It usually takes lots of time and effort. Sometimes wasting a week and not having the right solution is the best option. Of course, people will get stressed and mad. To remove stress, try to Vave login during some breaks.
Machine learning and operations research are two sophisticated advanced analytics technologies used in a wide range of solutions. And you need to know the specifics of each in order to correctly apply it in each of the business sectors.
Machine Learning (ML)
Machine learning is a tool with which you can find patterns in the input data and thus solve the problem. This technology is most successfully used in tasks where it is necessary to predict something. Such historical data is best suited for this, in which the algorithm finds a certain logic or pattern. ML is used in many industries, from medicine to different industries. It can be found in medicine. ML helps to recognize diseases based on the results of the ultrasound, X-ray, or MRI. Or with the help of this technology, mineral deposits are found.
Operations Research (OR)
Operations research activities aimed at the mathematical modeling of processes and the search for optimal solutions. This approach has long been used abroad. It is mainly used in large manufacturing companies, where a small change of one percent can bring a significant effect. This can be an optimization. With the help of OR there can be found the distribution of applications by a logistics company. It can save both the time of specialists and the costs of transport management. Or you can find the use of it in fertilizer production. The OR-based solution can respond faster to market changes and plan fertilizer production without manual calculations.
Main differences
In short, using ML, you can identify parameters and dependencies. But only OR will tell you the optimal solution. Here are three more differences. There are three types of analytics.
Descriptive. It gives an idea of what happened in the past or is happening in the present.
Predictive. This allows you to predict what to expect in the future.
Prescriptive. It helps you decide what needs to be done to achieve your business goals.
The fact is that ML is a predictive analytics tool with which you can process huge amounts of data and learn from them. The end result of machine learning work is a prediction that can be used to make a decision.
And OR refers to prescriptive analytics. It is used in a mathematical model. Such a model lists all the constraints of the process that you want to minimize or maximize,
and solve this model. The result of this approach will not be a forecast, but an optimal solution based on the available data and the input problem.
That is, with the help of machine learning, you can get a forecast for the next quarter’s sales. But with the help of operations research, you will learn what exactly needs to be improved in production in order to maximize the next quarter’s revenue.
Applications
We experience the impact of machine learning in everyday life. We love using speech and image recognition in a smartphone, and virtual assistants.
Operations research is widely used in various business areas to solve large-scale problems. It is used in production planning, staff scheduling, cargo routing, or distribution of goods or electricity.
The difference is that ML-based products are more consumer-oriented. Therefore, they are more popular and understandable. While OR-based tools are less visible. Because they are used primarily in business.
Adaptability
Both technologies work differently in the face of sudden changes. For example, if something suddenly changes in the operating environment, machine learning applications based on historical data may produce a less accurate forecast.
We saw this in 2019 during the pandemic. The demand for personal care products soared. It left the shelves in stores empty. So the companies were not immediately able to readjust to the new realities.
In the case of services based on OR, the situation is different. Since it uses a detailed mathematical model of the process, the data can be replaced with actual ones. And the result will correspond to the changed conditions.
In the airline industry, due to changing weather conditions or the need for maintenance, schedule disruptions often occur. Air France used mathematical optimization to schedule flights in such a way. Its flight delays and operating costs are significantly reduced.
Combined Approach
Definitely, a company can get a lot of benefits from a combination of ML and OR. For example, a mathematical model for decision-making can be enriched with a forecasting model. Then the decision will be even more optimal and balanced. Or take the optimization of the production schedule. Which we have already talked about.
You can create an ML model. It will take into account historical data and predict demand depending on the season and other factors. After, create an OR model. It will produce the optimal schedule for the production of products. So the combination of the costs of creation and storage, taking into account consumption, will be minimal. And many companies are already using this combined approach.
Amazon, for example, combines big data, machine learning, and operations research to optimize logistics. Google Maps uses ML to predict future traffic and OR to suggest the best path to a destination.
ML or OR?
Take off from the task. Machine learning and operations research fit different types of problems. Technologies using ML will help you predict something or draw a conclusion based on a lot of data. Predict consumer demand or diagnose equipment. And operations research will help you quickly make the best of many possible decisions. For example, to optimize the operation of transport or to allocate resources.
Pay attention to the data. For ML, high data quality is very important. The result directly depends on this. In the case of OR, a thorough knowledge of internal processes and the ability to build a mathematical model are important. The work of representatives of the departments involved in the processes. Also, the work of analysts and mathematicians is especially important here.
Focus on the result you want to get from technology. ML reveals parameters and dependencies. But the final decision on them is made by a person. OR gives the most optimal solution. It makes it clear what needs to be done to improve processes.
Use the power of synergy, or you don’t have to pick just one. Both of these technologies are not mutually exclusive, but complementary. They can be combined and create powerful services to solve the strategic and operational goals of the company.