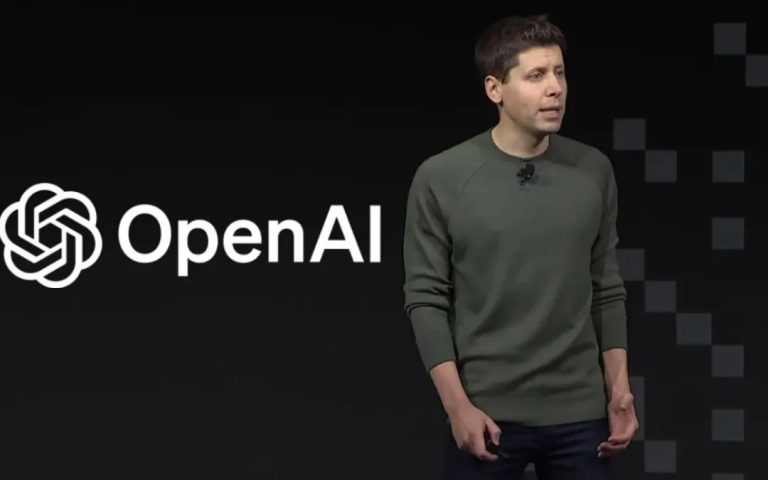
A glaring mismatch between internal and independent benchmark scores for OpenAI’s recently released o3 AI model is raising fresh questions about the company’s transparency and the reliability of industry claims.
While the discrepancy is not unprecedented, it underlines an uncomfortable truth that artificial intelligence, still sold on the promise of human-level reasoning and logic, is not yet ready to be trusted at face value.
When OpenAI introduced its o3 model in December, it was with no shortage of flair. During a live-streamed event, Chief Research Officer Mark Chen said the model could correctly solve just over 25% of problems in FrontierMath, a challenging benchmark designed to test mathematical reasoning at a level far beyond standard AI performance. The next-best model at the time managed just under 2%.
Register for Tekedia Mini-MBA edition 17 (June 9 – Sept 6, 2025) today for early bird discounts. Do annual for access to Blucera.com.
Tekedia AI in Business Masterclass opens registrations.
Join Tekedia Capital Syndicate and co-invest in great global startups.
Register to become a better CEO or Director with Tekedia CEO & Director Program.
“Today, all offerings out there have less than 2% [on FrontierMath],” Chen said. “We’re seeing [internally], with o3 in aggressive test-time compute settings, we’re able to get over 25%.”
The claim stunned much of the AI research community, who viewed it as a major leap in machine reasoning. But months later, as the o3 model finally reached public hands, the shine has dulled. Independent testing from Epoch AI, the group that created the FrontierMath benchmark, has found that the released version of o3 achieves a much lower score of around 10%, far below what OpenAI originally showcased.
Epoch acknowledged that several factors could explain the difference. Their testing used a newer version of the benchmark dataset, and the model evaluated was the production-grade o3 — not the more powerful internal version OpenAI likely used for its earlier tests. The compute settings, model scaffolding, and even the subset of questions were different.
“The difference between our results and OpenAI’s might be due to OpenAI evaluating with a more powerful internal scaffold, using more test-time [computing], or because those results were run on a different subset of FrontierMath,” Epoch noted in its statement.
In other words, OpenAI’s headline result was real — just not representative of the model the public can use.
Supporting this, the ARC Prize Foundation, which also tested o3 before its public release, confirmed that the production model is “a different model […] tuned for chat/product use,” and that all the available o3 compute tiers are smaller than the one originally benchmarked. That technical gap matters, as more computing typically enables better performance.
OpenAI did not refute Epoch’s findings. Instead, it offered a familiar rationale: the version of o3 now available is optimized for speed and practical use, not for peak benchmark performance. Wenda Zhou, a technical staff member at the company, said last week during a livestream that the production o3 was deliberately engineered to be more cost-efficient and responsive at the expense of benchmark scores.
“We’ve done [optimizations] to make the [model] more cost efficient [and] more useful in general,” Zhou said. “You won’t have to wait as long when you’re asking for an answer, which is a real thing with these [types of] models.”
Still, the shift from one model to another without clearly distinguishing between them in public-facing benchmarks has once again spotlighted the credibility gap growing in the AI industry.
While technically not a lie, OpenAI’s choice to showcase the best-case results of a high-performance internal model and then release a significantly dialed-down version plays into a broader pattern. In a rush to dominate headlines and capture market attention, AI firms often blur the distinction between what is achievable in theory and what is available in practice.
Meta recently admitted it had benchmarked one model but released another. Elon Musk’s xAI was accused of misleadingly promoting Grok 3’s benchmark charts. Even Epoch, now on the side of scrutiny, was previously criticized for not disclosing its funding from OpenAI until after the company unveiled o3.
The pattern is growing familiar: model scores are gamed, fine print is overlooked, and developers and the public are left navigating a fog of performance claims they can’t independently verify.
And yet, for all the frustration, this may be part of the necessary turbulence that comes with the evolution of an emerging field. AI is still learning to walk, and the companies building it are trying, sometimes awkwardly, to translate research breakthroughs into practical tools. The road from lab to real-world deployment is rarely smooth. Models are often re-engineered for usability, cost, and latency, which inevitably introduces performance tradeoffs.
The benchmark discrepancy around o3 is not just a technical note — it is also a reminder that artificial intelligence, at this stage, still straddles the line between promise and practicality. It reveals that, for now, trust in AI remains elusive. And perhaps more importantly, it reinforces the need for independent verification, clearer disclosures, and transparent model reporting standards.
But viewed from another lens, these stumbles can be seen as evidence of progress. The fact that researchers like Epoch can even test and compare these models in the open and that they are holding even the largest labs accountable is a sign of a maturing industry. The tension between public utility and internal performance may not disappear soon, but the pressure to bridge that gap is growing.
OpenAI appears to be aware of this dynamic. The company says newer variants like o3-mini-high and o4-mini already outperform the standard o3 on FrontierMath, and a higher-end model, o3-pro, is expected to launch in the coming weeks. If true, the company may once again make headlines — but with them, the same responsibility will follow: clarity, accountability, and respect for the people using these tools.
Ultimately, artificial intelligence is not failing, it is revealing how fragile trust can be when science and marketing collide. And in that, the discrepancy over a math benchmark becomes less about numbers and more about the story the AI industry chooses to tell about itself.
Until that story becomes more consistent, analysts believe that critical scrutiny will remain essential. While the evolution of AI will likely bring better models, faster tools, and more capability, the path forward must include the humility to admit — openly and early, when the technology doesn’t yet live up to its promises.