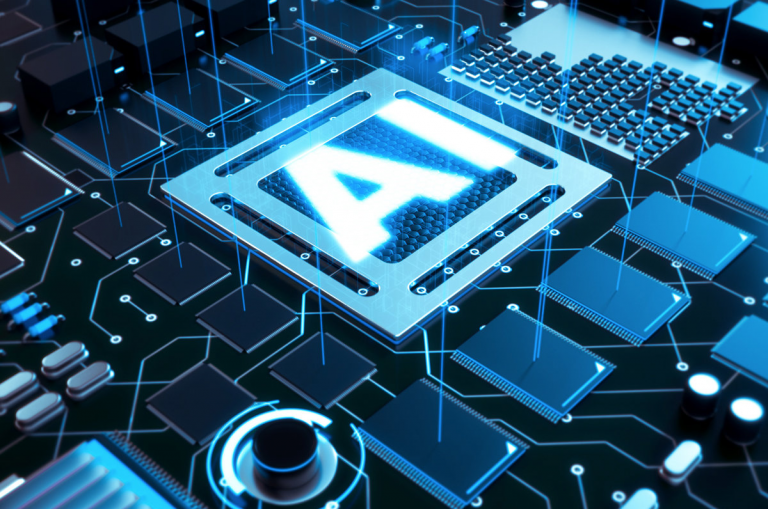
When you have data and have it in abundance, one of the major issues you must worry about is which variable is important in the implementation of your model. Another to worry about is what exactly to do with the data. Probably, another concern is how is my data creating value?
At the intersection of the above 3 worries lies the consumer, who either benefit from your intelligent use of your data or otherwise. And to realize value, customers must ultimately benefit from the utilization of their data in analytics which is ultimately your aim as well.
I recently purchased a pair of shoes from Jumia. Apparently, I checked lots of designs before settling on one. I made my purchase and I was pleased and extremely satisfied with my choice. But guess what? Every webpage that I opened in the past weeks have Ads from Jumia displaying shoes to me.
Register for Tekedia Mini-MBA edition 18 (Sep 15 – Dec 6, 2025) today for early bird discounts. Do annual for access to Blucera.com.
Tekedia AI in Business Masterclass opens registrations.
Join Tekedia Capital Syndicate and co-invest in great global startups.
Register to become a better CEO or Director with Tekedia CEO & Director Program.
Really!!! I wonder, should I still be getting shoe Ads even after purchasing one? Are the ads supposed to communicate to me that I made a bad choice or what? Are they to allure me to buy more shoes or what? What is the likelihood of me buying more shoes than I have already bought? Could it be that Jumia neglected a very important variable while building their recommendations and Ads engine? Or why on earth should shoe ads from Jumia be displayed all over my screen?
This is a case I referred to as poor machine learning implementation. The data point that has probably been neglected is very important (variable) here. If I checked an item on your website and refused to make a purchase (data available to you), then you have all justification to bombard my web pages with Ads of same items. Else if I had made the purchase, why would you think I will buy more than one? Neglecting a variable in a model build-up can become the bane of a model that otherwise would have been extremely valuable.
This is so because when you neglect an independent variable with high explanatory power on your dependent variable, you run the risk of being told a different story from what can be regarded as the real story. Truth be told neither I nor any other person will make a repeat purchase of what we bought and have not even utilized yet. Ask the people who run such Ads, it takes them nowhere.
What if instead of Jumia parading before my eyes pair of shoes I already bought they try to parade before my eye things people likely buy in addition to a pair of shoes? Say wristwatch, belt, shirts, and so on that can be regarded as up-selling or what Amazon typically calls “readers who bought this book also bought this”. Now, that is an intelligent use of machine learning.
You know by the virtues of your data points that this person bought these items already and the next thing he is likely to buy is this based on what the likes of him or her bought from previous purchases. Intelligent use of data and machine learning algorithm starts from following common sense, yes, common sense. Tosin Shobukola once said “we should be data-informed not data-driven” while trying to emphasis the importance of common sense while being data intelligent.
Data strategy
Data is being heralded as the new oil for a reason and the publicity is not unfounded. Data as we know it is the anchor of the new face of the industrial revolution where artificial intelligence becomes what we interact with in everyday life and that drive supper efficiency. Data can be tricky and the need to get it right from the foundation is important. Oil requires processes and mining before value can be outputted from it, this also is required of data.
It is not okay to just churn out models, between Zeros and Ones of all machine learning models lies logic, philosophy and abstraction of human behaviours. You need to factor in this reasoning to get maximum value from your data analytics and machine learning endeavour.
A level of thinking in terms of how logical, what philosophical stance do the majority of this strata buy into, how can we reduce this human behaviour to the level of Zeros and Ones is always involved.
Wow wow Wow!!! Great article!