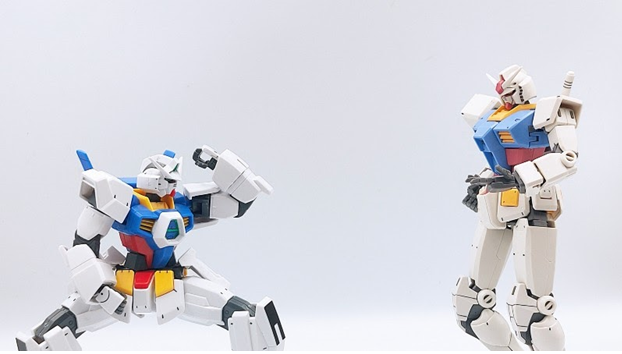
We may have seen cases where faces are misidentified on Facebook. But when algorithms misidentify the faces of crime suspects, it is not a joking matter. It is also not a laughing matter when face detection algorithms from well-known tech giant products misclassify faces of Serena Williams, Oprah Winfrey, and Michelle Obama as males.
In another note, On October 25, 2021, the Robomechanics research lab led by Professor Aaron Johnson of Carnegie Mellon University condemned the weaponization of mobile robots. According to Professor Aaron on his LinkedIn page:
“Robots should be used to improve people’s lives, not cause harm. As researchers driving the technological development behind these platforms, we have a responsibility to ensure that the technology is used in a constructive manner. As such, we will not work with companies that support the weaponization of their robots, whether autonomous or not. That is why last week my lab ended our partnership with Ghost Robotics and I resigned my (minor and unpaid) role as a scientific advisor to the company”
Register for Tekedia Mini-MBA edition 18 (Sep 15 – Dec 6, 2025) today for early bird discounts. Do annual for access to Blucera.com.
Tekedia AI in Business Masterclass opens registrations.
Join Tekedia Capital Syndicate and co-invest in great global startups.
Register for Tekedia AI Lab: From Technical Design to Deployment.
Photo Credit: IEEE Spectrum
The big question going forward is that should a robot be equipped for warfare and allowed to take kill or live decisions.
These are the current trending questions in robotics. They are, in fact, ethical questions and calls for concerns. They reveal very serious and genuine concerns. It gets worse when the robot is even more biased and this makes one ask, how should the autonomous vehicles be programmed to react in difficult or emergency situations? Who is to be blamed when an autonomous vehicle hits a person? The car manufacturer, owner of the vehicle or the (inactive driver) at the time of the accident? Should an autonomous vehicle have to choose in an unavoidable car accident, who should be hit.? An old person or a child? If you were faced with the Tunnel problem, what would you decide?
Tunnel Problem: You are traveling along a single-lane mountain road in an autonomous car that is fast approaching a narrow tunnel. Just before entering the tunnel a child attempts to run across the road but trips in the center of the lane, effectively blocking the entrance to the tunnel. The car has but two options: hit and kill the child, or swerve into the wall on either side of the tunnel, thus killing you. How should the car react?
Whether in autonomous cars or weaponized robots, ascribing responsibility for action during an emergency is a Gordian knot.
Photo Credit: becominghuman.ai
If humans are biased in the decision-making process, how then is the making of a human going to be less biased? If in our societies people of color are still protesting for cases of police racial profiling and discrimination, how then can we trust that a police robot equipped with warfare won’t be biased? Even if the police robot is not making decisions autonomously, the human remotely controlling it could be biased.
What should scientists and robotic engineers develop or research about? What happens when ethics clash with innovation. Should we sacrifice economics for ethics or vice versa?
Answers to many of these questions are going to determine the eventual acceptance and full deployment of autonomous systems (robots) in our society. We may not always blame the robot or its programmers for ethical issues as claims by car manufacturers say the self-driving car for example is not always the cause of the accident.
One of the original leaders of Google’s self-driving car project, Chris Urmson writes in 2015 about Google Driverless Car saying:
“If you spend enough time on the road, accidents will happen whether you’re in a car or a self-driving car. Over the 6 years since we started the project, we’ve been involved in 11 minor accidents (light damage, no injuries) during those 1.7 million miles of autonomous and manual driving with our safety drivers behind the wheel, and not once was the self-driving car the cause of the accident.”
The issue with ethics is not about whether robots are useful or not. We know they all are and can be put to very good use like in the surgical robots, farmlands robot, assistive robotic devices to mention but a few. In the 911 event, it’s the first time robots were deployed and used for rescue operations. Robot dogs are being used to deliver food in hostage situations, scout out a location where a gunman was located. The fear of the public, minority groups, and even researchers as regards robot dogs is the possibility of police violence, racism, and brutality, and unjustifiable killing which can come from weaponizing them, or the evolution of these robots from remote control to fully autonomous control and bias in the decision-making process. Thus, only until legal and ethical issues are solved, can we witness the full adoption of robots and autonomous vehicles in our society.
Algorithms today with the current state of development of AI and Robotics are still very much biased. David Berreby in a New York Times article wrote:
A robot with algorithms for, say, facial recognition, or predicting people’s actions, or deciding on its own to fire “non-lethal” projectiles is a robot that many researchers find problematic. The reason: Many of today’s algorithms are biased against people of color and others who are unlike the white, male, affluent, and able-bodied designers of most computer and robot systems.
Joy Buolamwin, founder of Algorithm Justice League in her Ted Talk also talks about algorithm bias and how a social robot could not detect her face in several instances. In one case, she had to borrow her white roommate’s face to finish the assignment. Later, working on another project as a graduate student at the M.I.T. Media Lab, she resorted to wearing a white mask to have her presence recognized by a facial detection algorithm because the people who coded the algorithm hadn’t taught it to identify a broad range of skin tones and facial structures. According to Joy, algorithms with bias can travel as fast as it takes to download files from the internet.
Another scenario by Professor Chris S. Crawford at the University of Alabama says:
“I personally was in Silicon Valley when some of these technologies were being developed,” he said. More than once, he added, “I would sit down and they would test it on me, and it wouldn’t work. And I was like, You know why it’s not working, right?”
Thus, there must be regulation and legislation and of course, full transparency before the public can fully accept and trust robots.
Robots are not just biased by nature; it starts with the algorithms that power these robots. Algorithms are also not self-written but written by humans. That is why solving bias really starts with people as opined by Joy in her TedTalk
Bias can lead to injustice which can be intentional or unintentional. Robert Julian-Borchak Williams was wrongfully accused by a facial detection algorithm of stealing in Detroit and he got arrested, spending hours in police custody and days in court trying to expunge his identity from a crime he didn’t commit.
The majority of cases of Bias come from the type of training data being used in the AI algorithm. Does the data have representatives in the whole sample population that will use the technology? If a facial detection algorithm is not trained with images of black men, such technology will not correctly identify a black man’s face. A machine learning algorithm will not make correct predictions or assumptions on information that is not accessible to it. Therefore, the training data must really represent the true population and not a skewed subset.
But beyond solving skewed dataset problems, a diverse engineering team will help in checkmating individual blind spots and biases.
Prof Chris Crawford says:
The long-term solution for such lapses is “having more folks that look like the United States population at the table when technology is designed. Algorithms trained mostly on white male faces (by mostly white male developers who don’t notice the absence of other kinds of people in the process) are better at recognizing white males than other people.
No matter how novel or innovative a technology is, how such a product will be used and who the end-users will use it matters a lot. A case in point here is the weaponization of robot dogs. A diverse training dataset representative of the true population, diverse team of different ages, gender, races, and ethnic groups including minority groups and multidisciplinary teams of engineers, lawyers, philosophers, psychologists, etc will be a game-changer in solving the challenge of bias in AI and robotics. Teachers and research lead also need to teach research methods and mentor students on identifying bias and solving the problem in their assignments and projects. This is why we now have several support and advocacy groups now like BlackInRobotics, BlackInAI etc
Importantly, minority groups need to step up their game and be part of the conversation. More students need to specialize and be subject matter expert in STEM, contribute to research and be part of the discussion since according to a West African adage, you cannot be absent from the meeting and complaint of not getting the share of the cake. African groups need to also develop a dataset that would represent their population and solve their challenges. Several works are being done along this line, but more work obviously needs to be done.
I will close with the words of Joy again, she says: “Thinking about the social impact of our biases matters. We need to create technology that works for all of us and not just some of us”
Hafeez Jimoh is a Power BI and Excel Trainer, Data Analyst and Robotics Researcher. You can connect with me on LinkedIn or twitter