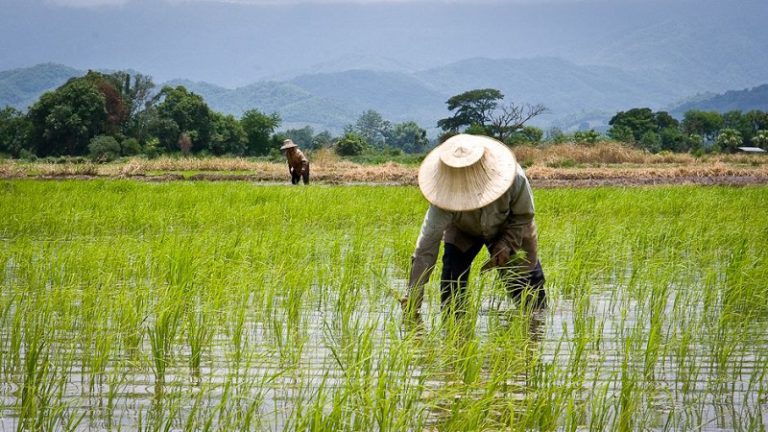
At Zenvus Smartfarm, one of our services is aggregating data and selling them via subscriptions to commodity traders as they optimize their commodity prediction algorithms. But we also make it possible for African farmers using our zPrices and zMarkets solutions to have the capacity to predict the future so as to position them to competitively price produce.
The core of our technology is a data forecasting engine which takes structured and unstructured data to predict future pricing patterns. We feed data from our platforms and combine our deep understanding of the continent to create the future. Our goal is to help farmers have a picture of where price is moving so that they can plan when to sell or hold.
The Zenvus engine is a deep mathematical model involving calculus, time series, analytics, and statistics. Upon development, we have tested in previous data with success rate of 80%-98%. We developed many statistical models and then took composites where necessary to come up with the best optimal results. The models, without the mathematics to avoid distractions, are as follows:
Register for Tekedia Mini-MBA edition 18 (Sep 15 – Dec 6, 2025) today for early bird discounts. Do annual for access to Blucera.com.
Tekedia AI in Business Masterclass opens registrations.
Join Tekedia Capital Syndicate and co-invest in great global startups.
Register for Tekedia AI Lab: From Technical Design to Deployment.
· Ensemble model: Ensemble modeling is the process of running two or more related but different analytical models and then synthesizing the results into a single score or spread in order to improve the accuracy of predictive analytics and data mining applications
· Self-exciting Threshold Auto-regressive (SETAR): SETAR models are applied to time series data as an extension of autoregressive models in order to allow for higher degree of flexibility in model parameters through a regime switching behavior.
· Bayesian vector auto-regressive: Bayesian vector autoregression (BVAR) uses Bayesian methods to estimate a vector autoregression (VAR). In that respect, the difference with standard VAR models lies in the fact that the model parameters are treated as random variables, and prior probabilities are assigned to them.
· Others models include Frequentist vector auto-regressive, Autoregressive integrated moving average(ARIMA), and integration of L’Hospital’s .
(If you did Further Mathematics in any decent secondary school in Nigeria (and indeed Africa), you would have mastered these constructs. One of the best topics in Further Mathematics used to be series and complex numbers. However, you will need a first year university Mathematics to understand the applications.)
The roadmap is to empower farmers so that right from their phones, they can text or app our database and receive predictions on the direction of price up to three months in the future. We feed locations of warehouses, volatility in regions, and many other factors to construct and train our algorithms. Using those factors, our tool predicts trajectory of future price. This is Machine Learning!
The Technology Components
Our technology is built with analytic heuristic capability to make sense of different data sets in varying formats. It has three nexus – Fusion, Discovery and Knowledge Engine
- Fusion: Collates your data and transform into human understandable form
- Discovery: Searches your data to unlock patterns
- Knowledge Engine: Provides insights from data.
The core engine architecture is as shown below.
Core Architecture
This engine was previously engineered in Milonics Analytics and was applied in some 2015 elections, as Milonics Citizen, in Nigeria including those of Kaduna State.
Testing the Models with Data
In this demo result below, we want to predict the price of ABS plastic. The key factors fed into the technology are World Business Confidence Index (an economic factor), WTI Crude Oil (a related commodity), butadene (related product), Economic Growth of G-7, etc. The results below are different predictions for the different models used. The last is the accuracy of the prediction which decreases as you move deep into the future. By the time you move to 11 weeks in the future, the confidence of prediction drops towards 80% which is bounded.
Ensemble Model
Ensemble Model (above). Other models are compared with it (below). The prediction is in sync across the models. With this historical data, the models are close with Week 2 but deviate into the future to about 82% in Week 11.
- Bayesian Model
- Arima model
- Frequentist Model
- SETAR
Model Accuracy Forecast
When you check the model accuracy data, you get the result below. Price prediction with historical data gets up to 98% accuracy in Week 1 but as you move deeper into the future, the result deviates to 82% at Week 11. This means that farmers can have decent insights on where pricing is going since typically 11 weeks will be optimal.
*Zenvus Smartfarm and Milonics Analytics are my companies. Some of the technologies are developed in Milonics Analytics and we’re re-using them in agriculture. Milonics Analytics customers include Nigerian Army, National Biotechnology Development Agency, United Bank for Africa Plc, Government of Botswana, UnityKapital Assurance Plc, etc.
Ndubuisi Ekekwe